CSC 458 - Predictive
Analytics I, Fall 2022, Assignment 5.
Assignment 5 due by 11:59 PM on Thursday December 15 via D2L.
Our "final
exam" class on 12/15 at 2 PM will be a work
session.
This is a crowd sourcing assignment. I have spent the majority of
my prep time this semester on CSC458 and CSC523 data science
projects. CSC220 Multimedia Programming students have mostly been
doing assignments from previous semesters, but for their final
assignment we are tackling something entirely new for a video
installation in Rohrbach Library in the spring. I just spent every
spare minute of Thanksgiving break and the week before prepping
their new code base / framework, so I am assigning my usual work
for this course to you.
Assignment 5 is a redo of one of Assignments 2, 3, or 4, using new
regressors and/or classifiers with new configuration parameters.
There is a 10% per day penalty for late assignments in my courses.
I need this by end of Friday 12/16 (late) to get grades in on
time.
1. Pick one of Assignments 2, 3, or 4. This is your choice.
Assignment
2 on numeric regression
Assignment
3 on data compression & discrete
classification
Assignment
4 on classification of nominal values and
time-series analysis
2. Replace all
regressors and/or classifiers in your assignment with new ones.
You can also reuse ensemble classifiers for which you make
significant changes to their base models and configuration
parameters.
For each new regressor or classifier that you select, find the
outline description in its pop-up More window like this:
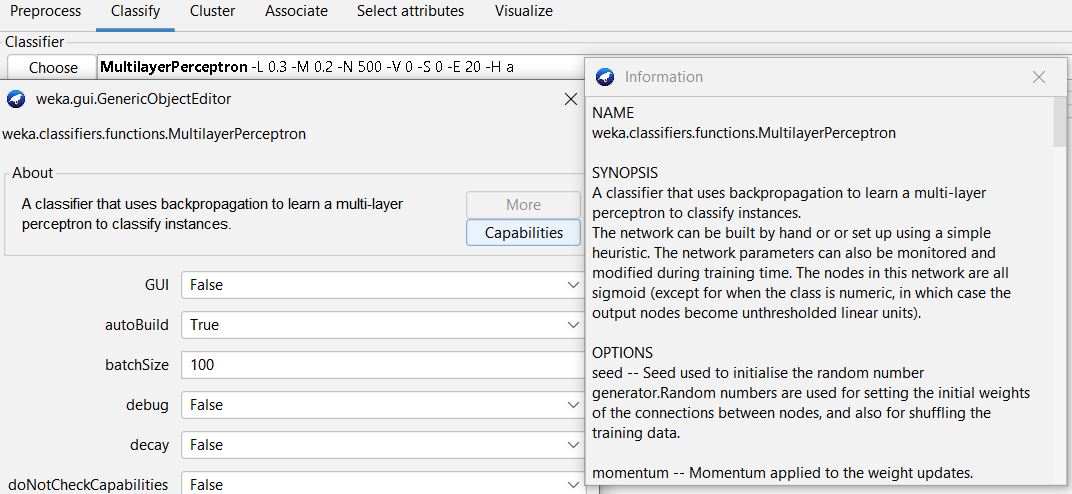
and paste the command line and More window output into the top of
your README.txt like this, preceding any Qn question. Do this for
all regressors and/or classifiers you select.
******************************************************************************************************
NAME
weka.classifiers.functions.MultilayerPerceptron
SYNOPSIS
A classifier that uses backpropagation to learn a multi-layer
perceptron to classify instances.
The network can be built by hand or or set up using a simple
heuristic. The network parameters can also be monitored and
modified during training time. The nodes in this network are all
sigmoid (except for when the class is numeric, in which case the
output nodes become unthresholded linear units).
OPTIONS
seed -- Seed used to initialise the random number generator.Random
numbers are used for setting the initial weights of the
connections between nodes, and also for shuffling the training
data.
momentum -- Momentum applied to the weight updates.
nominalToBinaryFilter -- This will preprocess the instances with
the NominalToBinary filter. This could help improve performance if
there are nominal attributes in the data.
hiddenLayers -- This defines the hidden layers of the neural
network. This is a list of positive whole numbers. 1 for each
hidden layer. Comma separated. To have no hidden layers put a
single 0 here. This layer definition will only be used if
autobuild is set. There are also wildcard values: 'a' = (attribs +
classes) / 2, 'i' = attribs, 'o' = classes , 't' = attribs +
classes.
validationThreshold -- Used to terminate validation testing.The
value here dictates how many times in a row the validation set
error can get worse before training is terminated.
GUI -- Brings up a gui interface. This will allow the pausing and
altering of the neural network during training.
* To add a node, left click (this node will be automatically
selected, ensure no other nodes were selected).
* To select a node, left click on it either while no other node is
selected or while holding down the control key (this toggles
selection).
* To connect a node, first have the start node(s) selected, then
click either the end node or on an empty space (this will create a
new node that is connected with the selected nodes). The selection
status of nodes will stay the same after the connection. (Note
these are directed connections. Also, a connection between two
nodes will not be established more than once and certain
connections that are deemed to be invalid will not be made).
* To remove a connection. select one of the connected node(s) in
the connection and then right click the other node (it does not
matter whether the node is the start or end: the connection will
be removed).
* To remove a node, right click it while no other nodes (including
it) are selected. (This will also remove all connections to it)
.* To deselect a node either left click it while holding down
control or right click on empty space.
* The raw inputs are provided from the labels on the left.
* The red nodes are hidden layers.
* The orange nodes are the output nodes.
* The labels on the right show the class the output node
represents. Note that with a numeric class the output node will
automatically be made into an unthresholded linear unit.
Alterations to the neural network can only be done while the
network is not running, This also applies to the learning rate and
other fields on the control panel.
* You can accept the network as being finished at any time.
* The network is automatically paused at the beginning.
* There is a running indication of what epoch the network is up to
and what the (rough) training error for that epoch was (or for the
validation set if that is being used). Note that this error value
is based on a network that changes as the value is computed.
(Also, whether the class is normalized will affect the error
reported for numeric classes.)
* Once the network is done, it will pause again and either wait to
be accepted or trained more.
Note that if the GUI is not set, the network will not require any
interaction.
normalizeAttributes -- This will normalize the attributes. This
can help improve performance of the network. This is not reliant
on the class being numeric. This will also normalize nominal
attributes (after they have been run through the nominal to binary
filter if that is in use) so that the binary values are between -1
and 1
numDecimalPlaces -- The number of decimal places to be used for
the output of numbers in the model.
batchSize -- The preferred number of instances to process if batch
prediction is being performed. More or fewer instances may be
provided, but this gives implementations a chance to specify a
preferred batch size.
decay -- This will cause the learning rate to decrease. This will
divide the starting learning rate by the epoch number to determine
what the current learning rate should be. This may help to stop
the network from diverging from the target output, as well as
improve general performance. Note that the decaying learning rate
will not be shown in the GUI, only the original learning rate. If
the learning rate is changed in the GUI, this is treated as the
starting learning rate.
validationSetSize -- The percentage size of the validation
set.(The training will continue until it is observed that the
error on the validation set has been consistently getting worse,
or if the training time is reached).
If this is set to zero, no validation set will be used and instead
the network will train for the specified number of epochs.
trainingTime -- The number of epochs to train through. If the
validation set is non-zero then it can terminate the network early
debug -- If set to true, classifier may output additional info to
the console.
resume -- Set whether classifier can continue training after
performing therequested number of iterations.
Note that setting this to true will retain
certain data structures which can increase the
size of the model.
autoBuild -- Adds and connects up hidden layers in the network.
normalizeNumericClass -- This will normalize the class if it is
numeric. This can help improve performance of the network. It
normalizes the class to be between -1 and 1. Note that this is
only internally, the output will be scaled back to the original
range.
learningRate -- The learning rate for weight updates.
doNotCheckCapabilities -- If set, classifier capabilities are not
checked before classifier is built (Use with caution to reduce
runtime).
reset -- This will allow the network to reset with a lower
learning rate. If the network diverges from the answer, this will
automatically reset the network with a lower learning rate and
begin training again. This option is only available if the GUI is
not set. Note that if the network diverges but is not allowed to
reset, it will fail the training process and return an error
message.
***********************************************************************************************
If you reuse an ensemble regressor or classifier from the earlier
assignment, change its base regressor or classifier.
3. Edit README.txt
At the top of the README.txt file list all of the regressor and/or
classifier changes you have made including exploration of
configuration parameters per above instructions.
Rewrite each README Qn question as needed and answer it. Some
questions may not fit your new models, or you may think of better
questions. In those cases just rewrite the Qn&A to explain
something that you discovered.
At the start of each Weka output Paste that you do, for example
accuracy and error measures, precede them by pasting these lines
from the top of that Weka test execution.
=== Run information ===
Scheme:
weka.classifiers.functions.MultilayerPerceptron -L 0.3 -M 0.2 -N
500 -V 0 -S 0 -E 20 -H a
Relation:
F2022Assn3Keepers-weka.filters.unsupervised.attribute.Remove-R46-47
Instances: 226
Attributes: 45
Those lines show me exactly what regressor or classifier you ran
to get that result.
I will deduct points if those lines are missing.
4. Turn assignment file(s) into D2L by the due date.
Have a good winter break!